My research spans Human-Computer Interaction, Artificial Intelligence, Software Engineering, Education, and Empirical Evaluation, where I develop innovative strategies, theories, visualizations, and prototypes tailored to user needs. Through a rigorous, iterative process—(1) conducting empirical studies to identify user needs and challenges, (2) developing theoretical frameworks to address these gaps, (3) designing tools that embody these frameworks, and (4) evaluating both the tools and the theories through confirmatory studies—I aim to create impactful, user-centered solutions. Employing mixed methods, my work integrates qualitative and quantitative approaches to design, assess, and investigate interactive systems, user needs, and tool adoption. Ultimately, my research provides practical solutions for professionals while revealing insights into user behavior and strategies, inspiring advances in programming environments, methodologies, and algorithms that shape the future of interactive systems.
Research Projects:
Conversational Agents to Facilitate Programming 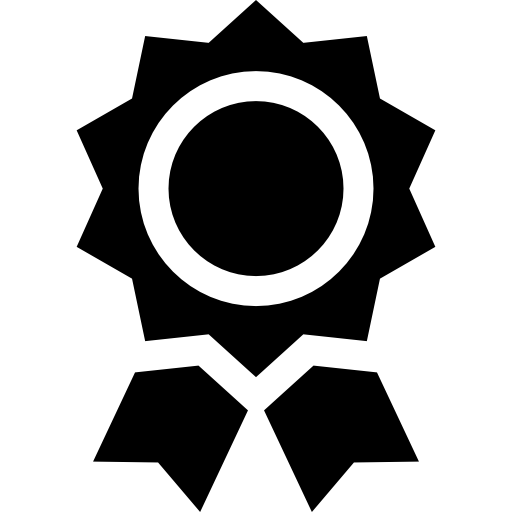
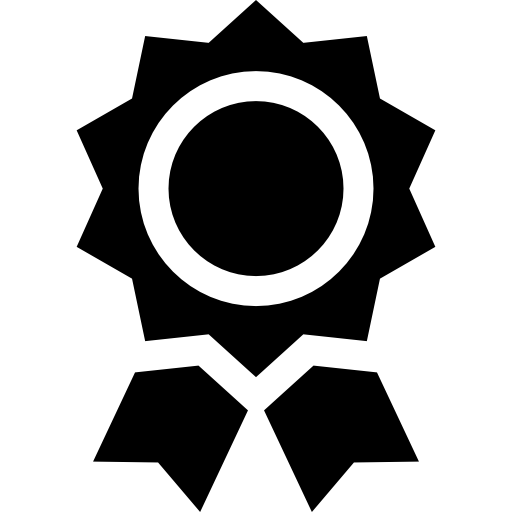
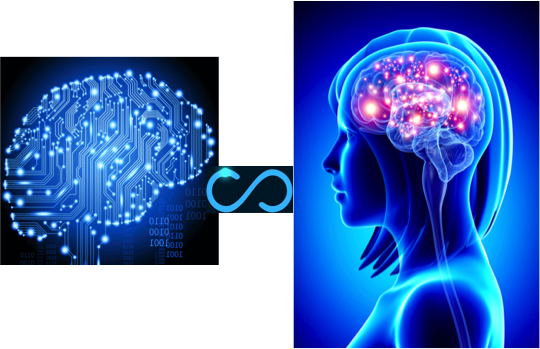
The goal of this research is to bring the benefits of pair programming to programmers by replacing a programmer with partner agents. This project aims to create a partner agents that is non-judgemental of gender, ethnicity, social and economic status and creates a symbiotic relationship to harness characteristics of humans (domain knowledge, creativity and innovation) and machines (automated techniques) to create powerful and efficient solutions.We are developing a paradigm called Pair Buddy to foster pair programming between the programmer and agents. The proposed research will gather and analyze rich fine-grained qualitative findings that further the state of knowledge about the programmers' collaboration with agents and inform the design guidelines for future collaborative pedagogical software and curriculum. These guidelines will inform the design of the Pair Buddy paradigm. Supported by (NSF CAREER Award, Honorable Mention Award)
Utilizing Agents' Collective Foraging Behavior 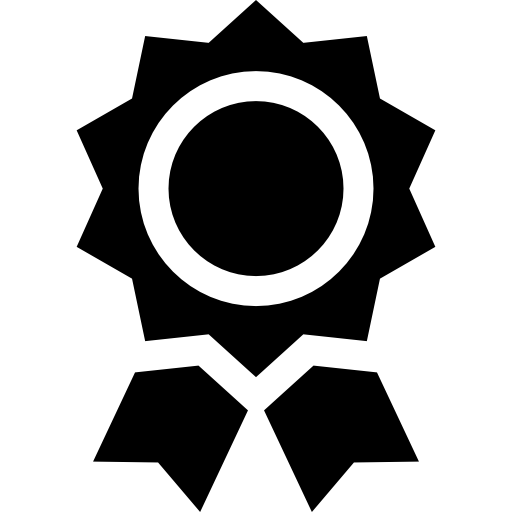
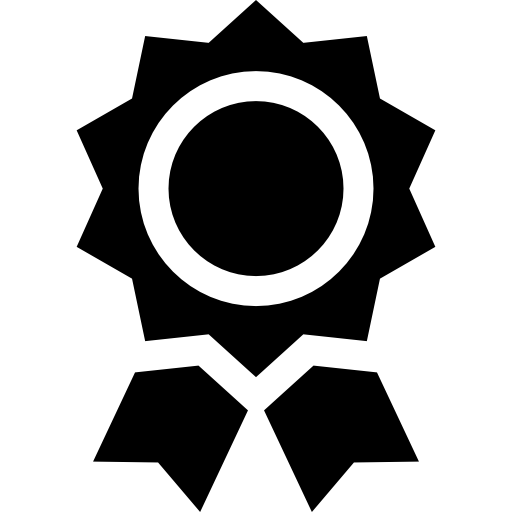
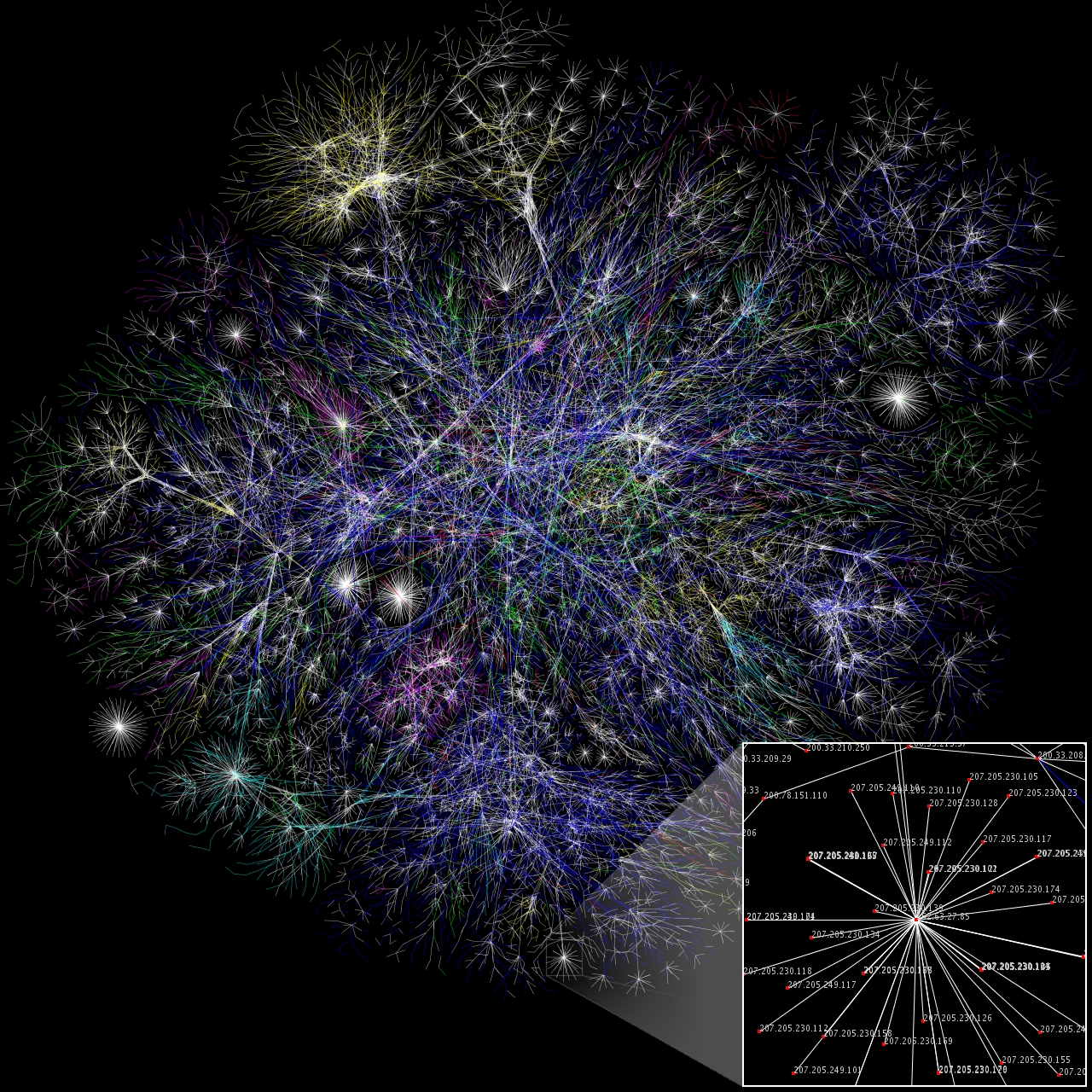
Collaborative software development allows collective intelligence but has the core problem of how to optimize the sorting and analysis of a vast array of “information fragments” in order to produce “optimal information” that is meaningful and applicable to a specific goal. We will investigate the use of the past collective information seeking behaviors of individual developers, specifically experts, to reduce the overhead of finding relevant information for new developers working on similar tasks. This research is inspired from the collective intelligence patterns among animals. Most creatures in the wild have developed ways to communicate information between individuals/groups when performing optimal foraging tasks. For example, ants use pheromone trails and stridulation, while honeybees use the celebrated waggle dance to recruit others to a food source. Similar to this behavior, we believe that developers also leave traces of their intelligence (e.g., commit logs), and their foraging behavior among artifacts (within OSS and on the web) that can be captured and utilized. To understand the information seeking and foraging behavior of individuals, we are utilizing Information Foraging Theory - a theory of information seeking that has been applied successfully to diverse domains such as web, interfaces and programming. Supported by (U.S. Airforce YIP Award, Best Paper Award)
Understanding and Modeling Gender Inclusive Collaborations
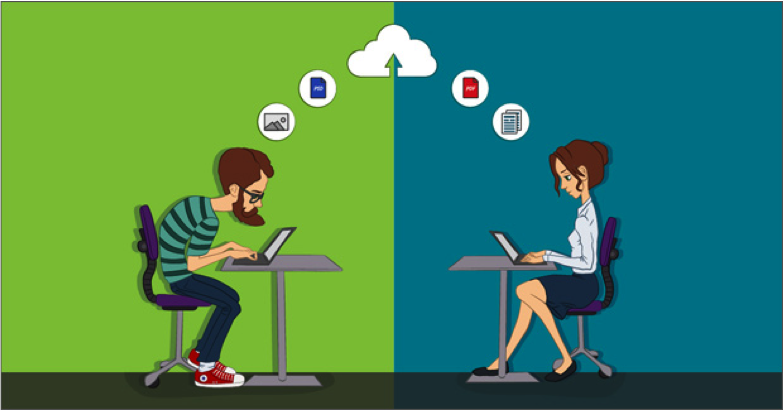
Although gender differences in a technological world receive significant research attention, much of this research and practice aims at how society and education can impact the success and retention of women in computer science. The possibility of gender biases within software, however, has received almost no attention. A method called GenderMag is developed. GenderMag (Gender-Inclusiveness Magnifier) is an inspection method for software practitioners to evaluate and create a gender-inclusive problem-solving software. Currently, we are working on extending the GenderMag method to GenderMag-Collab for supporting pair-programming. We also plan on developing tool to broaden the participation in computer education. Additionally, we are interested in characterizing brain neural response in the same and mixed genders pairs during collaboration on problem-solving task. Finally, utilizing machine learning to identified the differences in brain neural responses in the same and mixed gender dyads during problem solving. This research is in collaboration with Laureate Institute for Brain Research.
Assessment of Programmers' Socio-Technical Skills 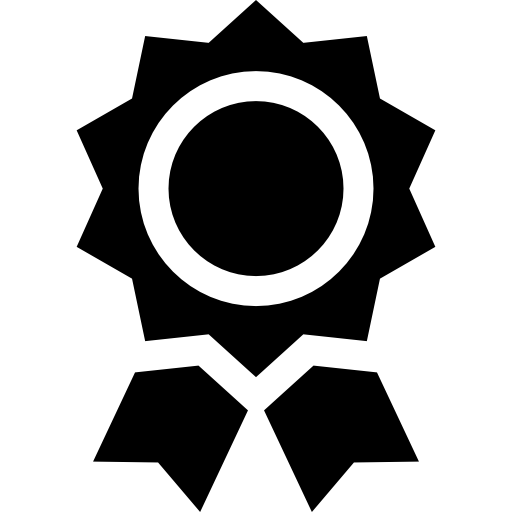
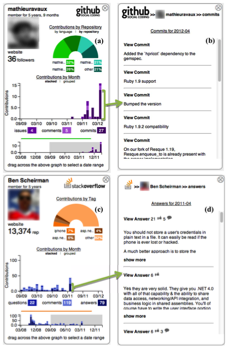
Managers are increasingly using online contributions to make hiring decisions. However, it is nontrivial to find relevant information about candidates in large online, global communities. Potential employers, as well as recruiters, are increasingly using the history of public contributions to locate suitable candidates, filter applicants for a position, or inform interview interactions. Literature suggests that both types of information (technical and social skills) are important when assessing developers. We designed Visual Resume to aggregate activity traces across two different types of peer production sites: a code hosting site (GitHub) and a technical Q&A forum (Stack Overflow). It aggregates developer activities across projects and languages to portray their technical and soft skills. More specifically, it extracts histories of commits, issues, comments, programming languages, and projects in GitHub. For Stack Overflow it groups data on answers, questions, comments, and tags. Aggregating activities across online communities can build a more accurate profile, since developers can contribute to multiple projects and forums. This also helps in comparison of contributions across sites as different sites can have different presentation styles. Our scenario-based, formative study found that participants appreciated the ability to compare candidates based on overall summaries, and to drill down to a particular contribution to assess its quality. ( Best Paper Award )
Understanding and Modeling Programmer's Explorations at File level 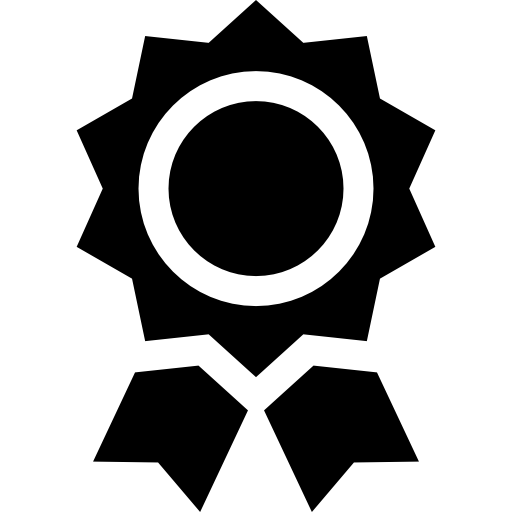
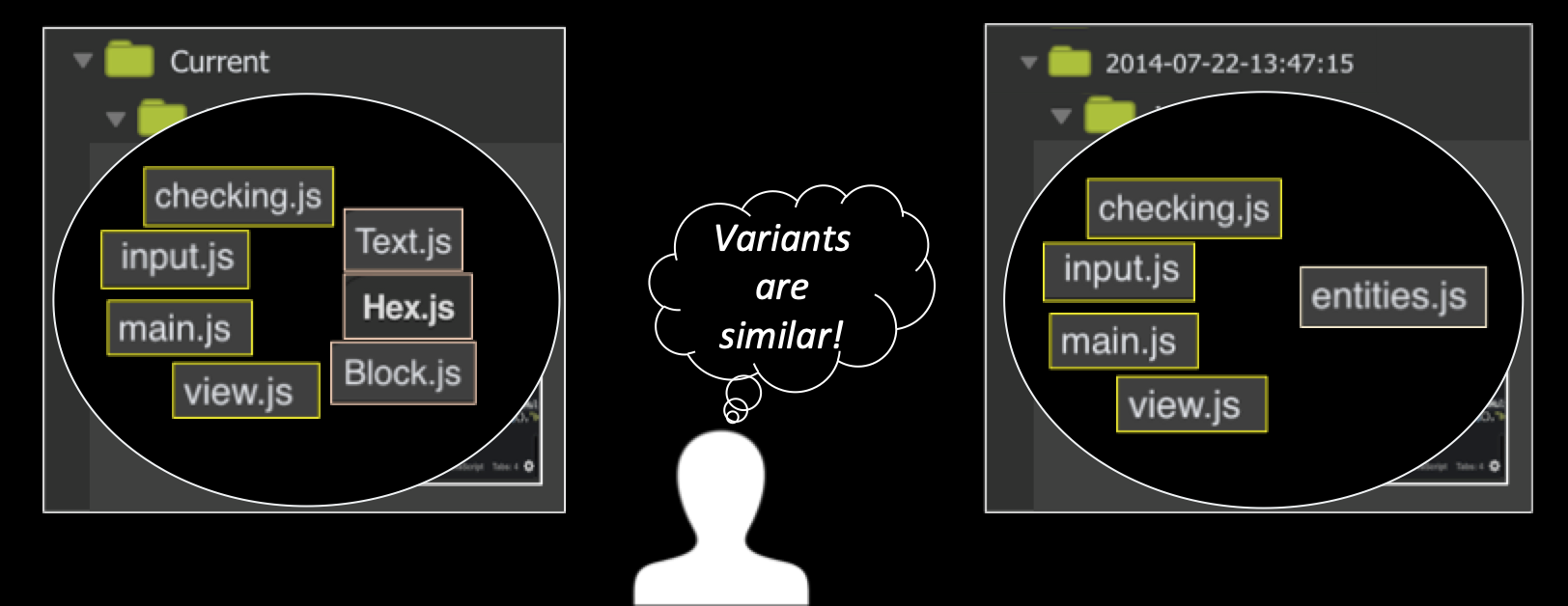
Computer-supported creative tasks—such as writing, graphic design, creating presentations, and some forms of programming— are often exploratory in nature. People often need to build upon and compare multiple ideas, fit them together and save the intermediate steps. Foraging among too many similar variants of the same artifact need more cognitive effort especially for no-vice programmers. We took a first step towards a theory of variation foraging through a qualitative empirical study investigating how novice programmers reuse variants. Based on our findings, we developed a predictive model, PFIS-V, a computational model of foraging that is able to account for multiple similar variants in an information space. Our empirical results show that PFIS-V is up to 25% more accurate than the previous programming computation model in predicting where a forager will navigate in a variationed information space. Based on these studies we found that if we account for complex information organized hierarchically we can increase the accuracy of finding variants and hence developed PFIS-H computational model. (Best Paper Award)
Mining Technical and Social Skills of Programmers
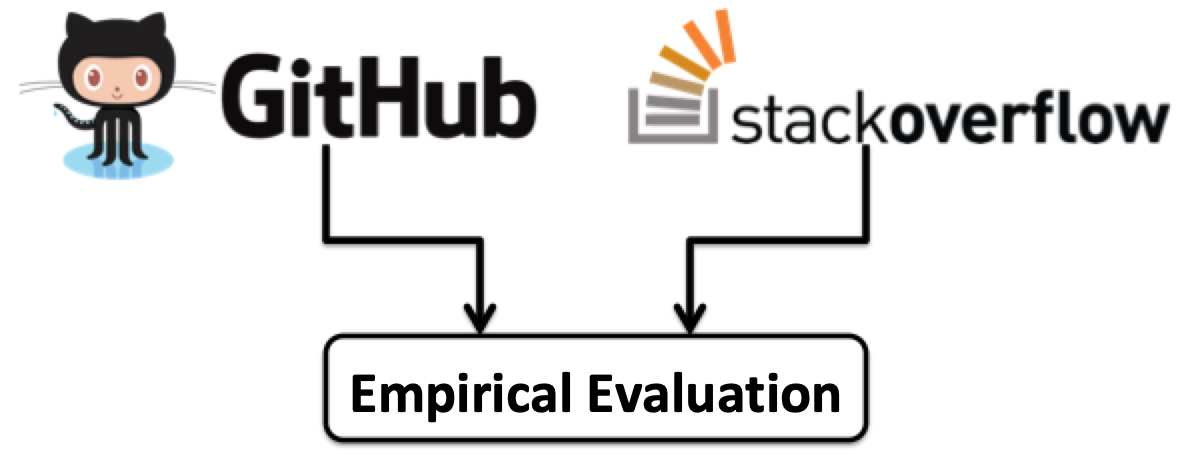
I am using social network analysis and deep machine learning techniques to understand the socio-technical behavior of programmers. For example, I am investigating how people learn code between their social and technical collaborations. I am also investigating the social behavior of the programmers when they move within and between social and technical sites. The understanding of these will help in designing tools for searching code, which programmers can trust and are based on their social interactions.
Semantic Clone Detection using Source Code Comments
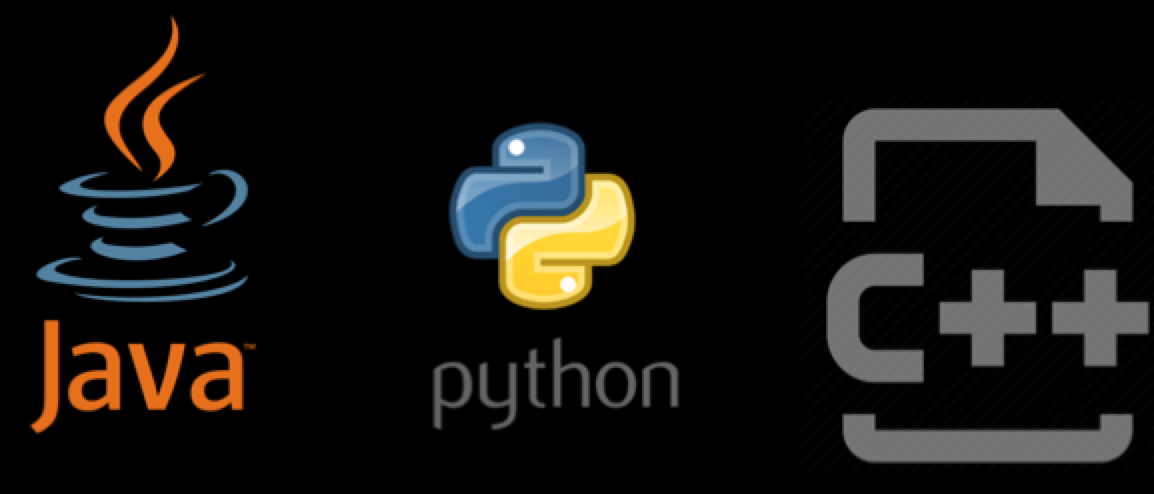
Programmers reuse code to increase their productivity, which leads to large fragments of duplicate or near-duplicate code in the code base. The current code clone detection techniques for finding semantic clones utilize Program Dependency Graphs (PDG), which are expensive and resource-intensive. PDG and other clone detection techniques utilize code and have completely ignored the comments - due to ambiguity of English language, but in terms of program comprehension, comments carry the important domain knowledge. utilized with LDA and are equivalent to sophisticated PDG based techniques. One approach would be using comments with LDA to detect clone sets at the file level, as this process is less resource-intensive, and applying PDG based code detection techniques at the function level.
Supporting Problem Solving
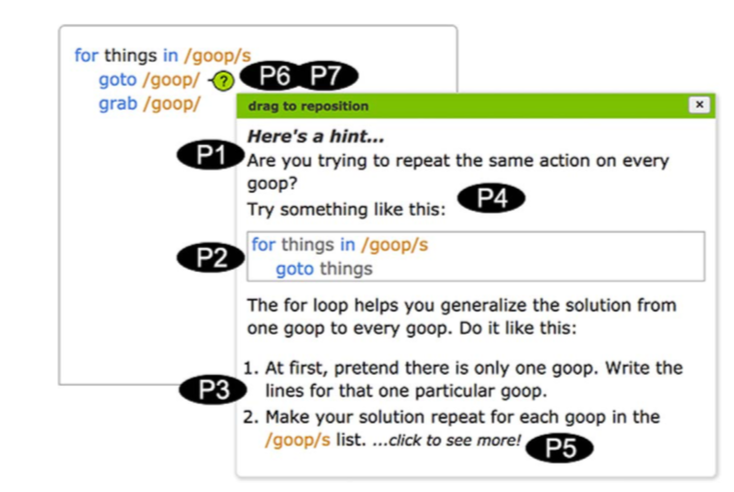
We have developed a theory-based approach called the “Idea Garden” that considers how end-user (non-professionals) programmers generate ideas when learning programming concepts for the first time based on Minimalist theory. It uses an integrated, just-in-time combination of scaffolding for problem-solving strategies, and for programming patterns and concepts. We have developed a generalized architecture to facilitate the inclusion of Idea Gardens into other systems. The Idea Garden approach has considered how EUPs generate ideas when learning programming concepts for the first time based on the diversity of EUPs' problem-solving situations. Hence, the Idea Garden supports EUP environments by providing context-sensitive advice, mini-patterns and problem-solving strategies that help them learn in the context of their own activities. We empirically investigated our prototype in three studies: a formative think-aloud study, a pair of summer camps attended by 42 teens, and a third summer camp study featuring a different environment attended by 48 teens. Our results showed that participants required significantly less in-person help than in a previous camp to learn the same amount of material in the same amount of time. Our studies showed the effectiveness of the Idea Garden's principles and support for different contexts in helping EUPs solve the programming problems that get them “stuck”—across a diversity of problems, information processing and problem-solving styles, cognitive stages, tasks, host IDEs, programming languages, and people.
Supporting Exploratory Programming (Ph.D. Dissertation)
It includes understand the foraging behavior of end user programmer while performing exploratory programming tasks such as program exploration, program understanding, verification and debugging in context of variations. My research has supported exploratory programming at different levels; namely, workspace, file, and online-repositories. My initial research was part of the Exploratory Programming project, a collaboration involving Carnegie Mellon University, Oregon State University, University of Nebraska-Lincoln, and University of Washington. We supported AppInventorHelper to support exploratory programming at file level and Pipes Plumber to support exploratory programming at the workspace level.
Supporting Debugging in Web Based Distributed Programming Environments: The web is a wild content rich platform and finding mechanisms to effectively and efficiently access information on it can be challenging. Creating applications that optimize information access and utilize web-based distributed programming involves aggregating heterogeneous web APIs distributed across different platforms. Building such programs is challenging as they contain software and hardware dependencies not represented in traditional programming environments. My own analysis of a large corpus (51,468) of (Yahoo! Pipes) mashup code and execution logs showed that more than 64.1% of mashups contained bugs primarily caused by changes in the sources' data. To provide better automatic detection of bugs, I created a classification scheme of bugs as Intra-module (bugs that occur within a module) and Inter-module (bugs that involve interactions between modules) bugs. Based on these classifications, an anomaly detector that can automatically detect bugs was implemented.
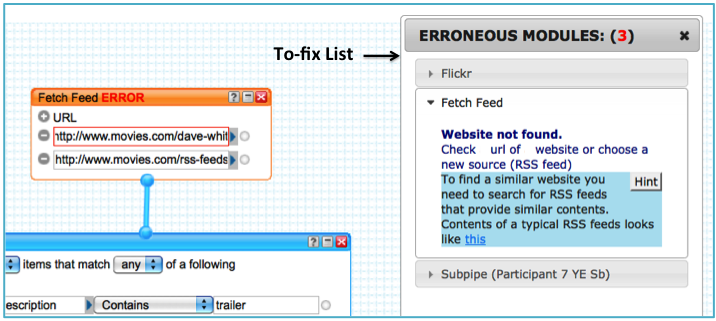
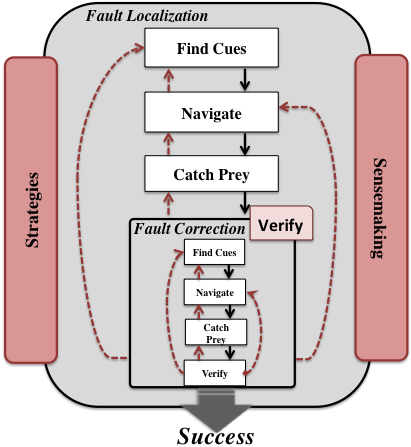
Using Information Foraging Theory (IFT) to understand the foraging behaviour while Web Based Distributed Programming Environments: To understand the foraging behavior of end-user programmers in the context of debugging mashups we used IFT. In IFT theory a predator (end-user programmer) forages for prey (bugs, while finding or fixing) by following cues (e.g., labels on links) in patches (e.g., web pages, IDEs). We contributed to the theory by creating a model that allowed us to refine our understanding of the debugging behavior of EUPs by separately focusing on the localization and correction of faults.
Click here to find details on my Ph.D. Dissertation.